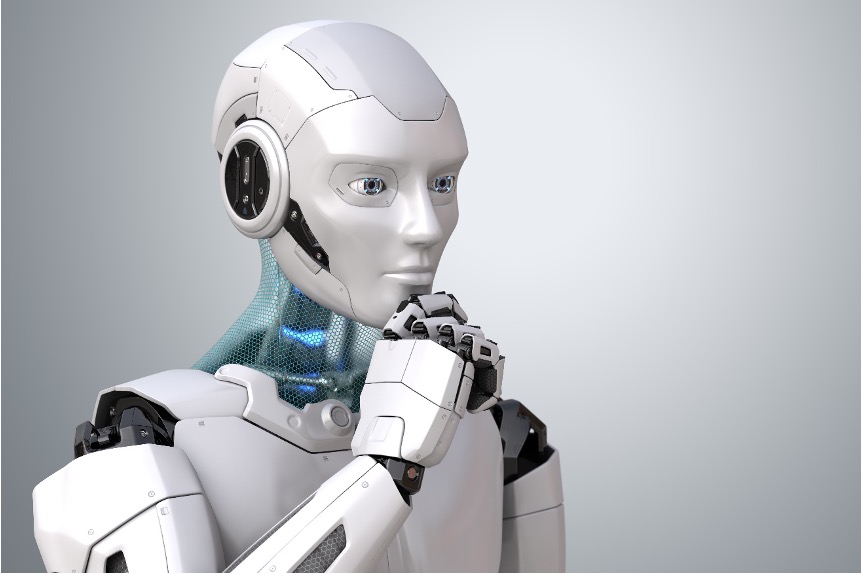
Thank you for your interest in the DCL Student Symposium! The Symposium is an annual event which brings together members of the Georgia Tech community working in the area of systems and controls to network and share their research. The Symposium is entirely organized by DCL students, and it is our hope that connections between students working in labs across the Institute will be forged at this event. We look forward to seeing you on Friday, April 18rd at the Symposium!
– Organizing Committee DCL Student Symposium 2025
Agenda
09:00 – 09:15 | Opening Remarks |
09:15 – 09:45 | Keynote Speaker I – Dr. S. T. Maguluri |
09:45 – 10:30 | 2-minute rapid talks |
10:30 – 11:30 | Student Poster Session + Coffee Break |
11:30 – 12:00 | Keynote Speaker II – Dr. Y. Chen |
12:00 – 13:00 | Lunch |
13:00 – 13:30 | Keynote Speaker III – Dr. S. Kousik |
13:30 – 14:00 | Keynote Speaker IV – Dr. L. Gan |
14:00 – 14:10 | Coffee Break |
14:10 – 14:45 | Faculty Panel: “The future of decision-making and control” |
14:45 – 15:45 | Student Spotlight Talk I |
15:45 – 16:00 | Coffee Break |
16:00 – 17:00 | Student Spotlight Talk II |
17:00 | Closing Remarks |
KEYNOTE TALK I – DR. SIVA THEJA MAGULURI (09:15 – 09:45)
“Seminorms, Stochastic Approximation, and Average Reward Reinforcement Learning“
Abstract
In the last few years, Reinforcement Learning has seen tremendous successes in a variety of engineering problems. Many of these problems involve finding the optimal policy of an underlying stochastic control problem where the objective is to minimize the long-run average cost. In this talk, I will present sample complexity guarantees for TD-Learning and Q-Learning in such settings. In contrast to the discounted cost that is more extensively studied in the literature, the average reward problem involves solving the Bellman equation that does not have a contraction property. However, it involves a contraction with respect to a seminorm. In this talk, we introduce seminorms, present stochastic approximation algorithms to solve for the ‘fixed points’ of operators under seminorm contraction property, and provide finite-sample convergence bounds. Due to the generality of these tools, they have applicability beyond RL.
KEYNOTE TALK II – DR. YONGXIN CHEN (11:30 – 12:00)
“Safety Assurance of Stochastic Systems“
Abstract
Safety is a critical requirement for real-world systems, including autonomous vehicles, robots, power grids and more. Over the past decades, many methods have been developed for safety verification and safe control design in deterministic systems. However, real-world applications often involve not only worst-case deterministic disturbances but also stochastic uncertainties, rendering deterministic methods insufficient. In this talk, I will present an effective framework that addresses this challenge by decoupling the effects of stochastic and deterministic disturbances. At the heart of this framework is a novel technique that provides probabilistic bounds on the deviation between the trajectories of stochastic systems and their deterministic counterparts with high confidence. This approach yields a tight probabilistic bound that is applicable to both continuous-time and discrete-time systems. By leveraging
this bound, the safety verification problem for stochastic systems can be reduced to a deterministic one, enabling the use of existing deterministic methods to solve problems involving stochastic uncertainties. I will demonstrate the effectiveness of this framework through several safety verification and safe control tasks.
KEYNOTE TALK III – DR. SHREYAS KOUSIK (13:00 – 13:30)
“Safety and performance in robotics: why not both?”
Abstract
Recent advances in deep learning have revolutionized the field of robotics. In particular, we are seeing a massive expansion in robot motion planning and control techniques that leverage data collected by teleoperating robots. However, these learned representations typically have no explicit notion of safety, instead relying on implicit safety in the training data. In this talk, we discuss two approaches for imbuing such learning-based methods with safety. First, we present Reachability-Aided Imitation Learning (RAIL), where we use a traditional safety filter in concert with a multimodal neural network policy and find that there is occasionally a surprising increase in task completion due to the safety filter pushing the policy towards more performant modes. Second, we discuss an approach for Neural Piecewise Affine Reachability Computation (NeuralPARC), where we directly perform reachability computations through a neural network motion planner; this allows us simultaneously to make strict guarantees on both collision avoidance and goal reaching, demonstrated in the extreme case of an autonomous vehicle drift parking maneuver. The key takeaway from this talk is that, with careful systems design, we can decouple the traditional tradeoff between safety and performance to get the best of both.
KEYNOTE TALK IV – DR. LU GAN (13:30 – 14:00)
“Toward Interpretable, Efficient, and Scalable Robot Perception”
Abstract
Recent advances in foundation models have opened new opportunities for general-purpose, robot-agnostic perception systems. While these models offer strong generalization and robustness, they often overlook valuable robot-specific priors that can enhance both performance and interpretability. In this talk, I will present recent efforts from my group to develop interpretable, efficient, and scalable robot perception systems by leveraging the structure and experience of specific robots. First, I will present a series of works on state estimation for legged robots that exploit their kinematic structures and morphological symmetries. Next, I will introduce approaches to legged robot navigation by learning terrain traversability from robot experience. Finally, I will briefly discuss a scalable, continuous semantic mapping pipeline designed to support large-scale, multi-robot deployments.
Faculty Panel: “The future of decision-making and control” (14:10 – 14:45)
STUDENT SPOTLIGHT TALK LIST
- Varkey M John: “Deception in Learning of Games: A Closed-Loop Stackelberg Game Analysis”
- Trent Schreiber: “Structural Model Updating with the P-RD Algorithm”
- Zishun Liu: “Safety Verification of Stochastic Systems via Probabilistic Tubes”
- Bhavini Jeloka: “Learning Large-Scale Competitive Team Behaviors with Mean-Field Interactions”
- Daniel Butterfield: “MI-HGNN: Morphology-Informed Heterogeneous Graph Neural Network for Legged Robot Contact Perception”
- Yun-Feng Lo: “Controlled Sensing for Phase-Shift Keyed Coherent States Discrimination”
- Chih-Yuan (Frank) Chiu: “Credit-based vs. Discount-based Congestion Pricing”
- Hoang Huy Nguyen: “Finite-Time Behavior of Erlang-C Model: Mixing Time, Mean Queue Length and Tail Bounds”
STUDENT POSTER LIST
- Amy Yao: “Hierarchical Path Planning for Agents with Computational Constraints using 3D Scene Graphs”
- Chih-Yuan (Frank) Chiu: “Credit-based vs. Discount-based Congestion Pricing”
- Belen Martin Urcelay: “Enhancing Human-in-the-Loop Learning for Binary Sentiment Word Classification”
- Maxwell Asselmeier: “Dynamic Gap: Safe Gap-based Navigation in Dynamic Environments”
- Luke Baird: “Runtime Assurance for Uncertain Systems from Interval Signal Temporal Logic”
- Cayetana Salinas: “What game are we playing? Inferring opponent intention in Stackelberg trajectory games”
- Junnosuke Kamohara: “RL-augmented MPC for bipedal locomotion under uncertainty”
- Nan Li: “Integrated Framework for Multi-Agent Task Allocation and Dynamic Path Planning”
- Daniel Butterfield: “MI-HGNN: Morphology-Informed Heterogeneous Graph Neural Network for Legged Robot Contact Perception”
- Trent Schreiber: “Structural Model Updating with the P-RD Algorithm”
- Brandon Ho: “Multi-agent Reinforcement Learning Motion Planner”
- Evangelos Psomiadis: “Hierarchical Semantic Path-Planning in 3D Scene Graphs”
- Lorenzo Ticozzi: “Hybrid Soft-Rigid Space Manipulators for On-Orbit Servicing”
- Zishun Liu: “Safety Verification of Stochastic Systems via Probabilistic Tubes”
LOCATION
The Symposium will take place in the Tech Square Research Building 1st floor auditorium area, on the right as you enter the building. The plenary sessions, student spotlight talks, and panel will take place in the auditorium. The poster session, lunch, and snack breaks will be located in the foyer directly outside the auditorium.
For questions regarding Symposium details, please contact Akash Harapanahalli at aharapan [at] gatech.edu.