October 16, 2020 – 03:15 PM
https://bluejeans.com/998437780?src=join_info
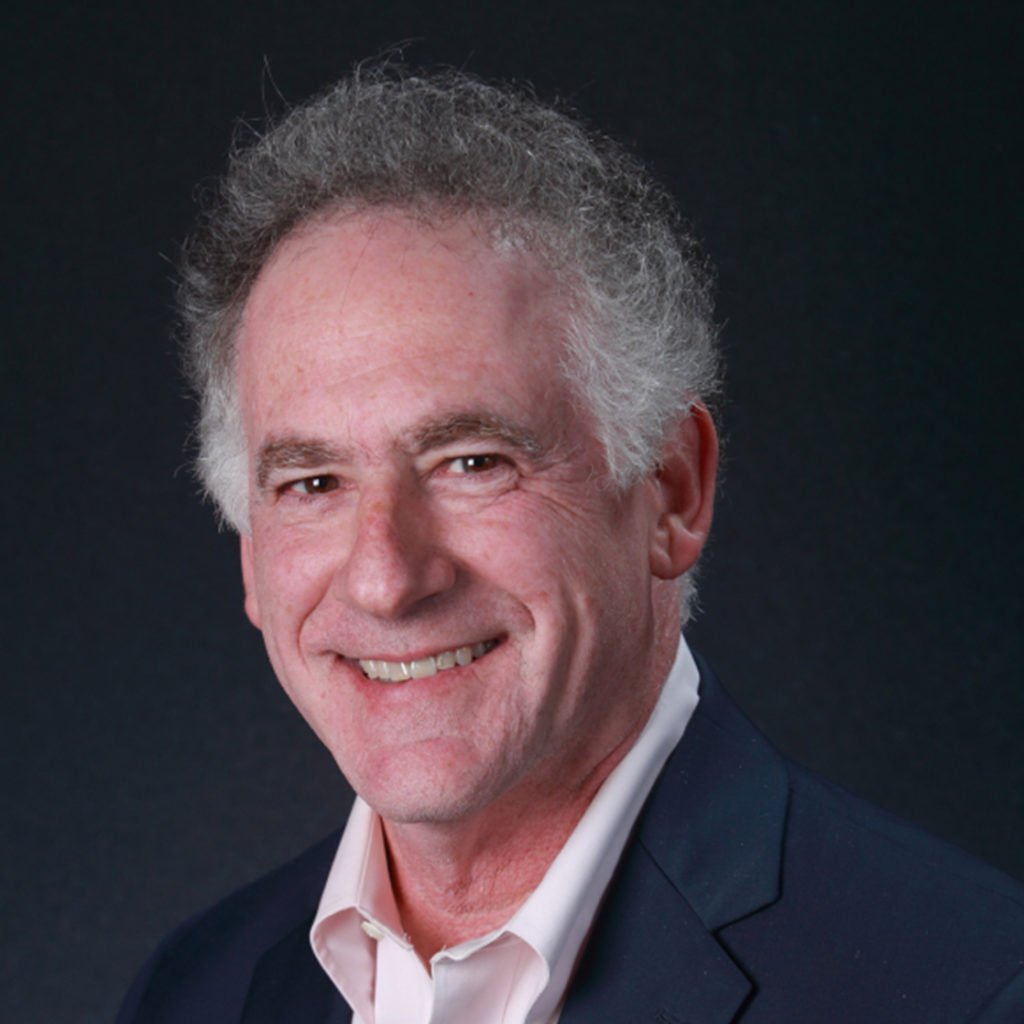
Paul K. Newton
University of Southern California
Abstract
I will introduce three problems in mathematical oncology all of which involve nonlinear dynamics and control theory. First, I will describe our work using Markov chain models to forecast metastatic progression. The models treat progression as a (weighted) random walk on a directed graph whose nodes are tumor locations, with transition probabilities obtained through historical autopsy date (untreated progression) and longitudinal data (treated) from Memorial Sloan Kettering and MD Anderson Cancer Centers. Then, I will describe our models that use evolutionary game theory (replicator dynamics with prisoner’s dilemma payoff matrix) to design multi-drug adaptive chemotherapy schedules to mitigate chemo-resistance by suppressing ‘competitive release’ of resistant cell populations. The models highlight the advantages of antagonistic drug interactions (over synergistic ones) in shaping the fitness landscape of co-evolving populations. Finally, I will describe our work on developing optimal control schedules (based on Pontryagin’s maximum principle) that maximize cooperation for prisoner’s dilemma replicator dynamical systems. As much as possible with the Zoom format, I hope the seminar will be interactive and a starting point for further discussions.
Biography
Professor Newton received his B.S. (cum laude) degree in Applied Mathematics/Physics at Harvard University in 1981 and his Ph.D. in 1986 from the Division of Applied Mathematics at Brown University. He then moved to the Mathematics Department at Stanford University to work as a post-doctoral scholar under J.B. Keller. He became Assistant (1987) and Associate Professor (1993) in the Mathematics Department at the University of Illinois Champaign-Urbana (UIUC) and at the Center for Complex Systems Research (CCSR) at the Beckman Institute. In 1993 he moved to the Aerospace & Mechanical Engineering Department and the Mathematics Department at the University of Southern California and was promoted to Full Professor in 1998. Trained as an applied mathematician, Professor Newton’s work focuses on developing mathematical models for nonlinear dynamical processes in continuum mechanics and biophysics, currently focusing mostly on mathematical oncology and systems biology. He has held visiting appointments at Caltech, Brown, Hokkaido University, The Kavli Institue for Theoretical Physics at UC Santa Barbara, and The Scripps Research Institute where he functioned as head of the mathematical modeling section of the NCI supported Physical Sciences Oncology Center (2009-2014). He is currently a Professor of Applied Mathematics, Engineering, and Medicine in the Viterbi School of Engineering, the Dornsife College of Letters, Arts and Sciences, the Norris Comprehensive Cancer Center in the Keck School of Medicine, and a founding affiliate member of the LJ Ellison Institute for Transformative Medicine of USC. He currently serves as Editor-in-Chief of the Journal of Nonlinear Science (SpringerNature).